Introduction
Given the volume of literature submitted to preprint servers daily, there is no feasible way for a human researcher to stay abreast of the state of a field at-large. BioRxiv, as a preprint server, exists as a public, fully transparent database of research published before peer-review. This comes with both advantages and drawbacks. For a researcher attempting to determine viable avenues of future research, bioRxiv is an indispensable resource. The volume of publiciation and short timescale place bioRxiv as the undisputed source for the most recent information. The second substantial benefit to using bioRxiv, is its API which allows for near seamless ingestion of data into programmatic tools. It should not be overlooked though, that bioRxiv is a preprint server. Papers submitted have been screened by a researcher with relevant expertise but have not been subjected to the full peer-review process. Because of this, warnings about reading overly into the findings of individual studies should be given extra consideration.
To meet the challenge of shrinking the search-space of academic literature, a wide array of techniques have been employed. At the most basic, the practice of citing sources creates a (theoretically) traceable lineage of research, each generation drawing on work done before. Papers sharing authors or keywords may be appropriately clustered more closely. Likewise, appearing in the same journal and being produced by the same institution are likely to be meaningful attributes linking two documents (fig.1) (Betts, Power and Ammar. 2019). This method benefits greatly from the rigorous way these attributes are typically collected. It is nearly unthinkable that a paper would be published in any repository without authorship or citations. While the specific metadata collected by each publishing company may vary somewhat, these attributes provide a valuable first step in mapping the search-space.
Related Work
No one algorithm can be said to dominate the fields of text clustering and classification. Due to the heterogeneity of language-based tasks, different models may be more appropriate for one workload over another. Traditional statistical methods such as term frequency – inverse document frequency (tf-idf) are often useful exploratory steps when handling relatively small datasets. More recently, deep-learning approaches are often favored for their ease of parallelization and ability to capture a greater amount of syntactic nuance.
A methodology following traditional statistical methods, was employed by Weisser et al (2020). Here, data cleaning consists of: duplicate removal, tokenization, stop-word and punctuation removal, language detection (dropping non-English results). After data have been cleaned, synonyms and hypernyms are mapped, and lemmatization back to root-words is performed (i.e. “read” and “reading” would be rendered equivalent.). Finally, the ratio of term frequency and inverse document frequency are calculated to encode the relevance of each term. After this point, a latent semantic analysis is used, wherein singular value decomposition is used to reduce the dimensionality of the data and attempt to control the amount of noise. K-means clustering can then be employed to group manuscripts.
For higher complexity tasks, traditional statistical methods such as TF-IDF and bag-of-words embeddings such as GLOVE have been largely abandoned in favor of neural-network-based models.
Lately, more sophisticated models have emerged as powerful tools for language-oriented tasks. The Bidirectional Encoder Representations from Transformers (BERT) (Devlin et al., 2018) model has risen to prominence since its publication as a state-of-the-art method for encoding not just individual words, but their bidirectional representations, preserving the context in which words appear. BERT embeddings have been shown to perform well in clustering tasks (Subakti, Murfi and Hariadi, 2022), and resist forming spurious connections (Tu et al., 2020).
Extending and challenging some of the progress made by the BERT model comes the cpt-text model by OpenAI. Cpt-text was trained using contrastive learning to generate an encoding which captures the level of similarity between two pieces of input text. From the resulting cosine similarity, documents can be plotted in 2D space.
Methods
i. Data
BioRxiv exists as a free, open pre-print repository which encompasses the bulk of biomedical publication. Because of BioRxiv’s focus on pre-publication documents, the daily volume of this dataset is very high. Model training is facilitated by the ease of use of the bioRxiv API, which provides abstracts and metadata in JSON format. Full text is available via an AWS bucket under a “requester pays” model and will be left out of the scope of this study. Following ingestion of data into Python, metadata is tokenized and padded.
ii. Choice of Algorithm
BERT, as an open-source package which can be run locally on consumer-level hardware, was selected over OpenAI’s CPT-text. CPT-text, by comparison must be run remotely on an OpenAI server, and returns upwards of 1024 tokens. For these reasons, among the highest performing encoders, BERT was selected for this project.
Extracting keywords from BERT models is an ongoing challenge with some plausible solutions. At the most basic, Pearson’s coefficients of correlation can be calculated for each term in the corpus. For a larger corpus such as used in this project, cosine similarity between given keywords and BERT sentence embeddings have proven to be a robust strategy as implemented in the KeyBert library (Sharma, P. Yingbo, L., 2019).
iii. Exploratory Analysis and Base Network
Dataset generation was carried out by use of the biorxiv API. The received data is JSON formatted for ease of use and was parsed into a dataframe for further processing. One year’s worth of submissions were downloaded and saved to a .CSV to be used as a training dataset. These publications represent the work of 36,474 unique authors from 2,954 institutions.
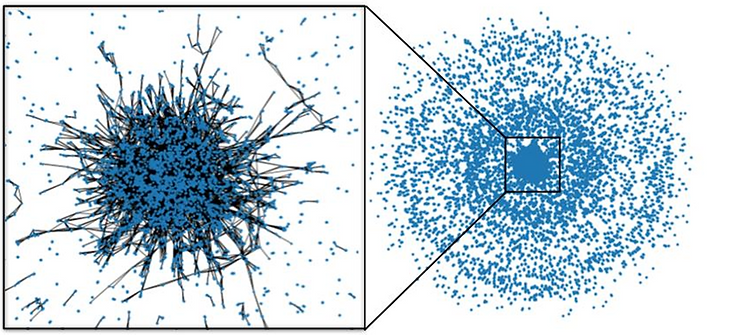
Incorporating the relatedness of the authors in the dataset, the NetworkX library was used to build a graph network of the authors listed on each paper. Nodes on the network represent an individual author, while edges represent a paper shared between the two authors. This visualization is largely randomly distributed. The number of authors in common is the only rule dictating the closeness of two authors. Even so, a clear cluster is present in the middle of the distribution. There is a large proportion of authors in the dataset wherein papers are much more closely connected than in the outlying points.
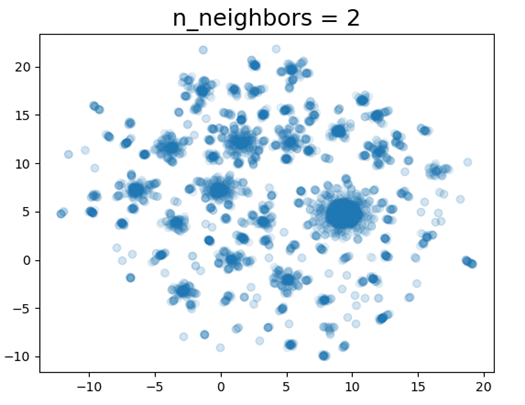
For a baseline of clustering and interpretability, UMAP was used on the raw BERT embeddings of each paper. The resulting map shows clear clustering. However, when Spearman’s coefficients were calculated against paper abstract term-frequencies, there are no clear trends.
Highest (left) and lowest (right) correlating key-words for component 1
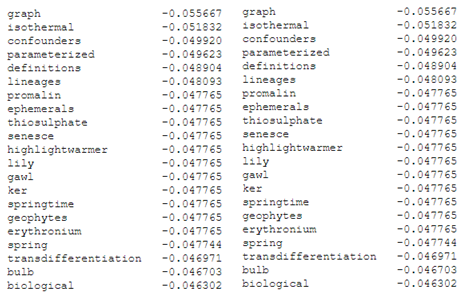
Highest (left) and lowest (right) correlating key-words for component 1
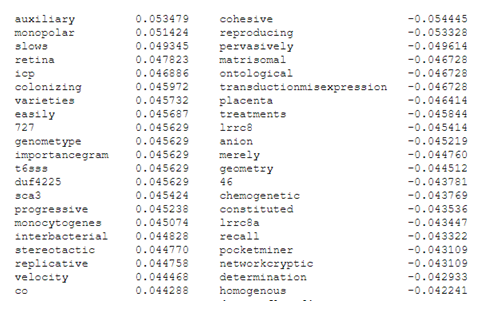
Autoencoder and Dimensionality Reduction
Each result’s title and abstract were next vectorized using BERT with a lexicon size of 1500. The generated encodings were concatenated to be passed to the autoencoder model for dimensionality reduction. The autoencoder consists of five encoder layers which narrow to 32 central nodes that constitute the latent dimension. The decoder precisely mirrors the encoder’s architecture. Layers are connected by hyperbolic tangent (tanh) activation. Training took place over four epochs, each iterating over the entire 1-year dataset. The corpus is then processed and the resulting embeddings are sent to umap for determination of similarity.
Results
The resulting manifold (fig n) maps the distance between each paper as determined by the autoencoder and UMAP. Calculating spearman’s coefficients between term-frequency embeddings and the eigenvalues produced by UMAP results in a transparent and relatively interpretable set of trends.
Highest (left) and lowest (right) correlating key-words for component 1
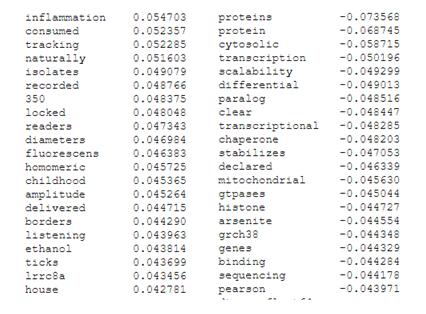
Highest (left) and lowest (right) correlating key-words for component 2
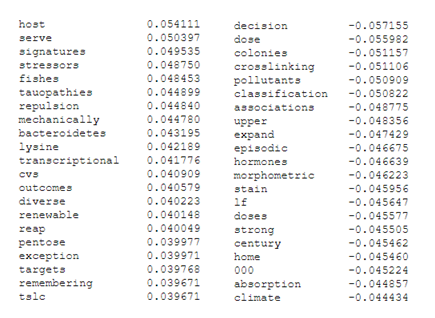
It appears likely that component 1 has captured the bulk of the difference between holistic and molecular methods. Keywords such as “childhood” and “inflammation” seem to closely track whole-animal research, while “histone”, “protein” and “binding” are typical of biochemical and molecular research. Component 2 is more difficult to parse, but may be capturing differences between in-vivo and wet-lab work (“host”, “fishes”, “lysine”), and in-silico or environmental work i.e. “decision”, “pollutants”, “associations”.

Further exploring this area, it may be useful to include a time component to the analysis. These data are heavily dependent on timescale and trends are likely to exist in both term-frequency and in the embeddings. These resulting embeddings have demonstrated the utility of further dimensionality reduction through autoencoders to produce transparent and interpretable results from large natural language datasets. Given a larger model or larger corpus of data, it may be possible to generate new insights into novel research directions. These results did not include the full texts of the articles. Given that full-article texts are a larger, richer body of data, one can predict that the resultant groupings would be still more indicative of underlying trends in scientific publication.
References
Betts, C., Power, J., and Ammar, W. 2019. GraphAL: Connecting the Dots in Scientific Literature. Association for Computational Linguistics. http://dx.doi.org/10.18653/v1/P19-3025
Devlin, J., Chang, M.W., Lee, K., and Toutanova, K. 2018 Bert: Pre-training of Deep Bidirectional Transformers for Language Understanding. Arxiv https://doi.org/10.48550/arXiv.1810.04805
Neelakantan A., Xu T., Puri R., Radford A., Han J. M., Tworek J., Yuan Q., Tezak N., Kim J. W., Hallacy C., Heidecke J., Shyam P., Power B., Nekoul T. E., Sastry G., Krueger G., Schnurr D., Such F. P., Hsu K., Thompson M., Khan T., Sherbakov T., Jang J., Welinder P., Weng L. 2022. Text and Code Embeddings by Contrastive Pre-Training. Arxiv. https://doi.org/10.48550/arXiv.2201.10005
Sharma, P., Yingbo, L. 2019. Self-supervised Contextual Keyword and Keyphrase Retrieval with Self-Labeling. Preprints.org https://doi.org/10.20944/preprints201908.0073.v1
Subakti, A., Murfi, H., Hariadi, N. 2022 The performance of BERT as data representation of text clustering. Journal of Big Data. https://doi.org/10.1186/s40537-022-00564-9
Tu, L., Lalwani, G., Gella, S., He, H. 2020 An Empirical Study on Robustness to Spurious Correlations using Pre-trained Language Models. MIT press direct. https://doi.org/10.1162/tacl_a_00335
Weisser, T., Sassmannshausen, T., Ohrndorf, D., Burgraf, P., and Wagner, J. 2020. A clustering approach for topic filtering within systematic literature reviews. Elsevier MethodsX. https://doi.org/10.1016%2Fj.mex.2020.100831
Leave a Reply